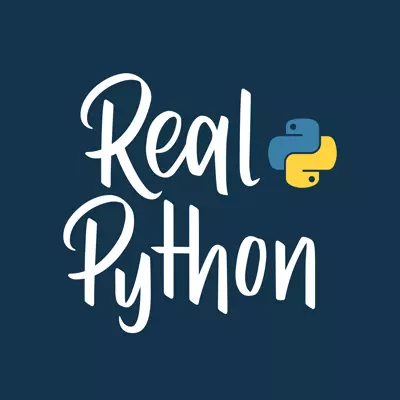
A weekly Python podcast hosted by Christopher Bailey with interviews, coding tips, and conversation with guests from the Python community. The show covers a wide range of topics including Python programming best practices, career tips, and related software development topics. Join us every Friday morning to hear what's new in the world of Python programming and become a more effective Pythonista.
Moving NLP Forward With Transformer Models and Attention
What’s the big breakthrough for Natural Language Processing (NLP) that has dramatically advanced machine learning into deep learning? What makes these transformer models unique, and what defines “attention?” This week on the show, Jodie Burchell, developer advocate for data science at JetBrains, continues our talk about how machine learning (ML) models understand and generate text.
This episode is a continuation of the conversation in episode #119. Jodie builds on the concepts of bag-of-words, word2vec, and simple embedding models. We talk about the breakthrough mechanism called “attention,” which allows for parallelization in building models.
We also discuss the two major transformer models, BERT and GPT3. Jodie continues to share multiple resources to help you continue exploring modeling and NLP with Python.
Course Spotlight: Building a Neural Network & Making Predictions With Python AI
In this step-by-step course, you’ll build a neural network from scratch as an introduction to the world of artificial intelligence (AI) in Python. You’ll learn how to train your neural network and make predictions based on a given dataset.
Topics:
- 00:00:00 – Introduction
- 00:02:20 – Where we left off with word2vec…
- 00:03:35 – Example of losing context
- 00:06:50 – Working at scale and adding attention
- 00:12:34 – Multiple levels of training for the model
- 00:14:10 – Attention is the basis for transformer models
- 00:15:07 – BERT (Bidirectional Encoder Representations from Transformers)
- 00:16:29 – GPT (Generative Pre-trained Transformer)
- 00:19:08 – Video Course Spotlight
- 00:20:08 – How far have we moved forward?
- 00:20:41 – Access to GPT-2 via Hugging Face
- 00:23:56 – How to access and use these models?
- 00:30:42 – Cost of training GPT-3
- 00:35:01 – Resources to practice and learn with BERT
- 00:38:19 – GPT-3 and GitHub Copilot
- 00:44:35 – DALL-E is a transformer
- 00:46:13 – Help yourself to the show notes!
- 00:49:19 – How can people follow your work?
- 00:50:03 – Thanks and goodbye
Show Links:
- Recurrent neural network - Wikipedia
- Long short-term memory - Wikipedia
- Vanishing gradient problem - Wikipedia
- Vanishing Gradient Problem | What is Vanishing Gradient Problem?
- Attention Is All You Need | Cornell University
- Visualizing A Neural Machine Translation Model (Mechanics of Seq2seq Models With Attention) – Jay Alammar
- Standing on the Shoulders of Giant Frozen Language Models | Cornell University
- #datalift22 Embeddings paradigm shift: Model training to vector similarity search by Nava Levy - YouTube
- Transformer Neural Networks - EXPLAINED! (Attention is all you need) - YouTube
- BERT 101 - State Of The Art NLP Model Explained
- How GPT3 Works - Easily Explained with Animations - YouTube
- Write With Transformer (GPT2 Live Playground Tool) - Hugging Face
- Language Model with Alpa (GPT3 Live Playground Tool) OPT-175B
- Big Data | Music
- OpenAI API
- 🤗 (Hugging Face)Transformers Notebooks
- GitHub Copilot learned about the daily struggle of JavaScript developers after being trained on billions of lines of code. | Marek Sotak on Twitter
- Jodie Burchell’s Blog - Standard error
- Jodie Burchell 🇦🇺🇩🇪 (@t_redactyl) / Twitter
- JetBrains: Essential tools for software developers and teams