Python Bytes is a weekly podcast hosted by Michael Kennedy and Brian Okken. The show is a short discussion on the headlines and noteworthy news in the Python, developer, and data science space.
Similar Podcasts
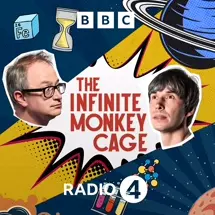
The Infinite Monkey Cage
Brian Cox and Robin Ince host a witty, irreverent look at the world through scientists' eyes.
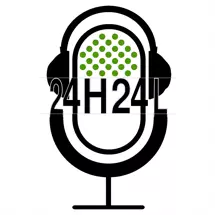
24H24L
Evento en línea, de 24 horas de duración que consiste en la emisión de 24 audios de diversas temáticas sobre GNU/Linux. Estos son los audios del evento en formato podcast.
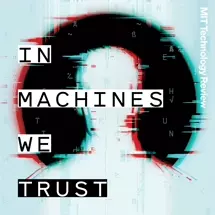
In Machines We Trust
A podcast about the automation of everything. Host Jennifer Strong and the team at MIT Technology Review look at what it means to entrust artificial intelligence with our most sensitive decisions.
#296 pip: Constrain your excitement
Watch the live stream: Watch on YouTube About the show Sponsored by the IRL Podcast from Mozilla Brian #1: Pip constraints files by luminousmen You can put some constraints on your dependencies with a constraints file. “Constraints files are requirements files that only control which version of a requirement is installed, not whether it is installed or not. “ Syntax is a subset of requirements.txt syntax but all the restrictions seem reasonable, considering must have a name can’t be editable can’t specify extras (that one is maybe slightly weird) You can put --constraint constraints.txt right at the top of your requirements.txt file or specify it on command line, pip install --constraint constraints.txt -r requirements.txt Or, my favorite, stick it at the top of requirements.in file. yes. pip-compile correctly handles constraints when generating requirements.txt. Example requirements.in --constraint constraints.txt typer constraints.txt click<8.1.3 Output from pip-compile requirements.in # # This file is autogenerated by pip-compile with python 3.10 # To update, run: # # pip-compile requirements.in # click==8.1.2 # via # -c constraints.txt # typer typer==0.6.1 # via -r requirements.in Michael #2: async-cache A caching solution for asyncio Quite simple but looks effective and flexible too Example: # TTL Cache from cache import AsyncTTL @AsyncTTL(time_to_live=60, maxsize=1024) async def func(*args, **kwargs): """ time_to_live : max time for which a cached result is valid maxsize : max number of results that are cached. if max limit is reached the oldest result is deleted. """ pass Brian #3: Organize Python code like a PRO Guilherme Latrova Yes, this is one author’s opinion. but… lots of great advice nothing too weird no tool recommendations Recommendations of note keep a src dir. A cool and simple reason: it keeps your source code together in alphabetized IDEs. file/module names: plural except for config, main, core, or similar slightly weird tangent that there are no files, there are modules. ok, whatever. Also talking about directories as main modules. odd. but ok. functions/methods should be verbs variables/constants should be nouns Class names should be singular, unless the class really represents a container The __name__ == "__main__" trick for modules. The __main__.py entry point trick for modules/packages so that -m mymodule does something. - Michael #4: keyring via Trent The Python keyring library provides an easy way to access the system keyring service from python. It can be used in any application that needs safe password storage. It’s also helpful in that it allows you to write your own backends. Useful for testing. Basically create a dummy keychain that stores to a pytest temp_path fixture instead of cluttering the real keychain. You could potentially write a backend to interact with any service such as 1Password. Extras Brian: I’m taking a class on FastAPI. The instructor is awesome! Also, editing some pytest course video. Michael: Gitlab, you alright?? Joke: from a dad-jokes repo Q: How do programming pirates pass method parameters? A: Varrrrarrrgs. Q: How do you get the code for the bank vault? A: You checkout their branch. "Unfortunately these jokes only work if you git them." Screw driver
#295 Flutter + Python GUI Apps?
Watch the live stream: Watch on YouTube About the show Sponsored by Microsoft for Startups Founders Hub. Michael #1: Faster routing for Flask & Quart Flask and Quart both utilise Werkzeug's HTTP route With the upcoming 2.2 release of Werkzeug this router will be significantly faster, with up to a factor of 5. Better for large sets of routes Micro-benchmarks are unaffected and are unlikely to show a speedup. Started with tree-based radix algorithm Moved to state machine algorithm because of wild cards Brian #2: Quarto: an open-source scientific and technical publishing system built on Pandoc suggested by Paul Mackenzie Power of Pandoc and Jupyter Build documents - html, pdf, word presentations - Revealjs, PowerPoint, Beemer websites books - html, pdf, word, epub journal articles - acm, plos, elsevier, acs, jss Publish GitHub pages, Netlify, … kinda related - Kindle to support ePub Michael #3: Flet UI via Mikael Honkala New and upcoming UI framework by Feodor Fitzner: flet. It has a very interesting stack - a Python client driving a Flutter front-end via a Go server. That sounds complicated, but the developer experience is incredibly simple. Installation is just pip install flet. Here's a quick and stupid but working sample: import time import flet from flet import Column, ElevatedButton, Page, Row, TextField def main(page: Page): text_field = TextField() def clear_field(event): text_field.value = "CLEARING" page.update() time.sleep(1) text_field.value = "" page.update() clear_button = ElevatedButton("Clear the field", on_click=clear_field) page.add(Row([Column([text_field, clear_button])], alignment="center")) page.update() flet.app(target=main) # If you run this, you get a native app window on Mac, Windows or Linux, looking something like this: While the example is simple, it shows the handling of an event, updating the UI, and even doing a little sleeping between the updates, without having to worry about threads and such. What's more important, if you change the last line to: flet.app(target=main, view=WEB_BROWSER) You get the exact same functionality, but as a web application in a browser, with support for multiple users and deep linking to different parts of the app. All without leaving the comfortable Python world, with its access to all Python libraries, and without having to learn 3 extra, completely different languages (yes, HTML, CSS and JavaScript). As this is Flutter, mobile support is in the works, after the basic UI functionality is all there. Check the project front page here: flet.dev Jump directly to the currently available controls: flet.dev/docs/controls Check couple of tutorials here: flet.dev/docs/tutorials Or read the plans for the mobile support here: flet.dev/blog/flet-mobile-strategy Brian #4: Building an authenticated Python CLI Project that uses click, rich, and OAth for using Twitter API Persistent authentication requests secret information from user using getpass and input Client ID, Client Secret, App name fetches bearer token from Twitter API stores token in netrc file I’m not familiar with netrc, so I don’t know if this is a good idea or not. So I figured I’d ask Michael Extras Michael: New course: Django - Getting Started Joke: Light touches kingdom
#294 Specializing Adaptive Interpreters in Full Color
Watch the live stream: Watch on YouTube About the show Sponsored by Microsoft for Startups Founders Hub. Michael #1: Specialist: Python 3.11 perf highlighter via Alex Waygood Visualize CPython 3.11's specializing, adaptive interpreter. 🔥 PEP 659 – Specializing Adaptive Interpreter Specialist uses fine-grained location information to create visual representations of exactly where and how CPython 3.11's new specializing, adaptive interpreter optimizes your code. Dark, rich colors indicate code with many quickened instructions (and, therefore, high specialization potential), while light, pale colors indicate code with relatively few specialization opportunities. Brian #2: tomli “A lil’ TOML parser” Fully compatible with TOML spec 1.0.0 This is the library that tomllib from Python 3.11 is based on, so great to use for Python 3.7-3.10 applications. We discussed Python 3.11 and PEP 680 on episode 273 Real Python has a great introduction for TOML in Python: Python and TOML: New Best Friends TOML as a config format, key-value pairs, data types using both tomli and tomllib Loading TOML documents into Python And like, writing, and updating toml docs programatically, which, although cool, I think the bulk of users can kinda skip over. But the the first 3 sections are an excellent reference. Tables are cool, with [name] and [name.subsection] syntax, as well as arrays of tables with [[name]] syntax. I didn’t know how to do that before this article. Michael #3: Pydantic V2 Plan via Douglas Nichols and John Thagen A very detailed plan Goal to have all this done by the end of October, definitely by the end of the year. Samuel Colvin take a sabbatical to work on this (sound familiar?) Some details highlighted by John: Moving the core logic to Rust to drastically increase performance (17x) https://pydantic-docs.helpmanual.io/blog/pydantic-v2/#performance Strict mode (something I've wanted for a long time): https://pydantic-docs.helpmanual.io/blog/pydantic-v2/#strict-mode Cleaning up required vs nullable: https://pydantic-docs.helpmanual.io/blog/pydantic-v2/#required-vs-nullable-cleanup Naming cleanup: https://pydantic-docs.helpmanual.io/blog/pydantic-v2/#model-namespace-cleanup This is a huge change, but overall it looks very promising for the community. It will likely require refactors by downstream users, so pinning pydantic using Poetry/pip-tools etc like always is a good idea. Many things have Pydantic at the core, so this matters, including: FastAPI Beanie SQLModel Pydastic … Brian #4: pikepdf a Python library for reading and writing PDF files Based on QPDF, which is written in C++. Features: Supports password protected PDFs Creates linearized ("fast web view") PDFs Integrates with Jupyter and IPython notebooks for rapid development Some cool uses copy pates from one PDF into another split and merge PDFs extract content replace content, such as replacing images, without altering the rest of the file. Documentation mentions that if you only want to write PDFs, consider other libs, such as reportlab. Extras Brian: pytest-check I’ve set up 2fa for my account, so now I have no excuse for not looking into feature requests and merge requests for pytest-check, other than like all the other things I’m doing. I don’t have data for the top 3,500 for the last 6 months, but there is a list of the top 5,000 for last 30 days. pytest-check is #1677 in the last 30 days. pytest is #72 on the same list. pydantic is #117 There are 57 pytest plugins that show up in the top 3,500 python packages. (packages that start with “pytest-”) pytest-check is #20 of those. I guess it’s time to do another top plugins episode of Test & Code. Joke: Error, OK, I’ll check the logs
#293 And if I pull this open source Jenga block...
Watch the live stream: Watch on YouTube About the show Sponsored by Microsoft for Startups Founders Hub. Special guest: Ashley Anderson Ashley #1: PSF security key giveaway for critical package maintainers Giving away 4000 2FA hardware keys Surely a team effort but I found it via @di_codes twitter (Dustin Ingram) links to previous talks on PyPI/supply chain security Interesting idea for helping with supply-chain vulnerabilities At least one dev pulled a critical package in response Previously: <add some links to prior discussions> I don’t have any critical projects Armin Ronacher has an interesting take Michael #2: PyLeft-Pad via Dan Bader Markus Unterwaditzer was maintaining atomicwrites More on how this relates to a project (Home Assistant) I wonder if PyPI will become immutable once an item is published Brian #3: FastAPI Filter Suggested and created by Arthur Rio “I loved using django-filter with DRF and wanted an equivalent for FastAPI.” - Arthur Add query string filters to your api endpoints and show them in the swagger UI. Supports SQLAlchemy and MongoEngine. Supports operators: gt, gte, in, isnull, it, lte, not/ne, not_in/nin Ashley #4: Tools for building Python extensions in Rust PyO3 pyo3 - Python/Rust FFI bindings nice list of examples people might recognize in the PyO3 README Pydantic V2 will use it for pydantic-core maturin - PEP 621 wheel builder (pyproject.toml) pretty light weight, feels like flit for Rust or python/Rust rust-numpy (+ndarray) for scientific computing setuptools-rust for integrating with existing Python projects using setuptools Rust project and community place high value on good tooling, relatively young language/community with a coherent story from early on Rust macro system allows for really nice ergonomics (writing macros is very hard, using them is very easy) The performance/safety/simplicity tradeoffs Python and Rust make are very different, but both really appeal to me - Michael #5: AutoRegEx via Jason Washburn Enter an english phrase, it’ll try to generate a regex for you You can do the reverse too, explain a regex You must sign in and are limited to 100 queries / [some time frame] Related from Simon Willison: Using GPT-3 to explain how code works Brian #6: Anaconda Acquires PythonAnywhere Suggested by Filip Łajszczak See also Anaconda Acquisition FAQs from PythonAnywhere blog From announcement: “The acquisition comes on the heels of Anaconda’s release of PyScript, an open-source framework running Python applications within the HTML environment. The PythonAnywhere acquisition and the development of PyScript are central to Anaconda’s focus on democratizing Python and data science.” My take: We don’t hear a lot about PA much, even their own blog has had 3 posts in 2022, including the acquisition announcement. Their home page boasts “Python versions 2.7, 3.5, 3.6, 3.7 and 3.8”, although I think they support 3.9 as well, but not 3.10 yet, seems like from the forum. Also, no ASGI, so FastAPI won’t work, for example. Still, I think PA is a cool idea, and I’d like to see it stay around, and stay up to date. Hopefully this acquisition is the shot in the arm it needed. Extras Michael: Python becomes the most sought after for employers hiring (by some metric) Ashley: PEP691 JSON Simple API for PyPI Rich Codex - automatic terminal “screenshots” Joke: Neta is a programmer
#292 Thursday, it's always a Thursday
Watch the live stream: Watch on YouTube About the show Sponsored by Microsoft for Startups Founders Hub. Brian #1: rich-codex by Phil Ewels suggested by Will McGugan “A GitHub Action / command-line tool which generates screen grab images of a terminal window, containing command outputs or code snippets.” Generate images from commands embedded in markdown files, like README.md, for example. Searches through markdown files for stuff like:  then runs the command, and generates the image. Can be done within a GitHub action Can also send code snippets or json to rich-cli, then generate an image. You can also have commands in a config file, Very easy to use, makes very professional looking images for documentation, that’s always up to date. Michael #2: Pydastic via Roman Right, by Rami Awar Pydastic is an elasticsearch python ORM based on Pydantic. Core Features Simple CRUD operations supported Sessions for simplifying bulk operations (a la SQLAlchemy) Dynamic index support when committing operations More on Elasticsearch here Brian #3: 3 Things to Know Before Building with PyScript by Braden Riggs Package indentation matters Local file access is possible. [HTML_REMOVED] - numpy - pandas - paths: - /views.csv [HTML_REMOVED] DOM manipulation has interesting conventions For buttons, you can include pys-onClick=”your_function” parameter to trigger python functions when clicked. For retrieving user input from within the [HTML_REMOVED] tag document.getElementById(‘input_obj_id’).value can retrieve the input value. And Finally pyscript.write(“output_obj_id”, data) can write output to a tag from within the [HTML_REMOVED] tag. Michael's Pyscript videos Python + pyscript + WebAssembly: Python Web Apps, Running Locally with pyscript Python iOS Web App with pyscript and offline PWAs Michael #4: disnake via Sean Koenig disnake is a modern, easy to use, feature-rich, and async-ready API wrapper for Discord. Features: Modern Pythonic API using async/await syntax Sane rate limit handling that prevents 429 errors Command extension to aid with bot creation Easy to use with an object oriented design Optimized for both speed and memory Quickstart Commands API Extras Michael: Scholarships for upcoming FastAPI + MongoDB live course Humble Bundle for Python 2022 Michael's crazy earbuds (UE Fits) Joke: Better than a wage increase
#291 Wait, you have how many licenses?!?
Watch the live stream: Watch on YouTube About the show Sponsored by us! Support our work through: Our courses at Talk Python Training Test & Code Podcast Patreon Supporters Michael #1: Python License tracker by Tom Nijhof/Nyhof Every package depends on other package with sometimes different licenses. Tom made a tool to find out what licenses you all need for a project: PyTest alone needs 4 different licenses for itself and its dependencies. Tensorflow is even worst Brian #2: undataclass Trey Hunner As a teaching aid, and to show how much dataclasses do for you, this is a module and an application that converts dataclasses to normal classes, and fills in all of the dunder methods you need. Example in app: from dataclasses import dataclass @dataclass() class Point: x: float y: float z: float Converts to class Point: __match_args__ = ('x', 'y', 'z') def __init__(self, x: float, y: float, z: float) -> None: self.x = x self.y = y self.z = z def __repr__(self): cls = type(self).__name__ return f'{cls}(x={self.x!r}, y={self.y!r}, z={self.z!r})' def __eq__(self, other): if not isinstance(other, Point): return NotImplemented return (self.x, self.y, self.z) == (other.x, other.y, other.z) Note on NotImplemented: It just means, “I don’t know how to compare this”, and Python will try __eq__ on the other object. If that also raises NotImplemented, a False is returned. The default is the above with @dataclass(frozen=True, slots=True) and adds the methods: fronzen=True gives you implementations of __hash__, __setattr__, __delattr__, __getstate__, __setstate__, Essentially raises exception if you try to change the contents, and makes your objects hashable. slots=True adds the line: __slots__ = (``'``x', '``y``'``, '``z``'``). This disallows adding new attributes to objects at runtime. See Python docs Trey wrote two posts about it: Appreciating Python's match-case by parsing Python code How I made a dataclass remover Turns out, this is a cool example for AST and structural pattern matching. Notes from the “how I made..” article: "I used some tricks I don't usually get to use in Python. I used: Many very hairy **match**-**case** blocks which replaced even hairier if-elif blocks A sentinel object to keep track of a location that needed replacing Python's **textwrap.dedent** utility, which I feel should be more widely known & used slice assignment to inject one list into another The ast module's unparse function to convert an abstract syntax tree into Python code” Michael #3: Qutebrowser via Martin Borus Qutebrowser is a keyboard-focused browser with a minimal GUI." It's Python powered Whats more important - doesn't force you to use it's Vim-based shortcuts, the mouse still works. But you usually don't need it: Because on any page, a keypress on the "f" key will show, you every clickable think and a letter combination to enter to click this. Brian #4: asyncio and web applications A collection of articles Quart is now a Pallets project P G Jones, maintainer of Quart and Hypercorn “Quart, an ASGI re-implementation of the Flask API has joined the Pallets organization. This means that future development will be under the Pallets governance by the Pallets maintainers. Our long term aim is to merge Quart and Flask to bring ASGI support directly to Flask. “When to use Quart?” “Quart is an ASGI framework utilising async IO throughout, whereas Flask is a WSGI framework utilising sync IO. It is therefore best to use Quart if you intend to use async IO (i.e. async/await libraries) and Flask if not. Don't worry if you choose the 'wrong' framework though, as Quart supports sync IO and Flask supports async IO, although less efficiently.” Using async and await, from Flask docs Flask has some support of async/await since Flask 2.0 But it’s still a WSGI application. “Deciding whether you should use Flask, Quart, or something else is ultimately up to understanding the specific needs of your project.” Should You Use AsyncIO for Your Next Python Web Application? Steven Pate A cool “brief history of Python web server interfaces” Discussion of the Python servers and frameworks for both WSGI and ASGI Recommendation: Do you need async? “… most people don’t. WSGI servers and frameworks are usually performant enough.” Extras Michael: Python Web Conf Talk: HTMX + Flask: Modern Python Web Apps, Hold the JavaScript browserosaurus Joke: Understanding JavaScript Joke: Where do you see yourself in 5 years?
#290 Sentient AI? If so, then what?
Watch the live stream: Watch on YouTube About the show Sponsored by us! Support our work through: Our courses at Talk Python Training Test & Code Podcast Patreon Supporters Special guest: Nick Muoh Brian #1: picologging From a tweet by Anthony Shaw From README.md “early-alpha” stage project with some incomplete features. (cool to be so up front about that) “Picologging is a high-performance logging library for Python. picologging is 4-10x faster than the logging module in the standard library.” “Picologging is designed to be used as a drop-in replacement for applications which already use logging, and supports the same API as the logging module.” Now you’ve definitely got my attention. For many common use cases, it’s just way faster. Sounds great, why not use it? A few limitations listed: process and thread name not captured. Some logging globals not observed: logging.logThreads, logging.logMultiprocessing, logging.logProcesses Logger will always default to the Sys.stderr and not observe (emittedNoHandlerWarning). Michael #2: CheekyKeys via Prayson Daniel What if you could silently talk to your computer? CheekyKeys uses OpenCV and MediaPipe's Face Mesh to perform real-time detection of facial landmarks from video input. The primary input is to "type" letters, digits, and symbols via Morse code by opening and closing your mouth quickly for . and slightly longer for -. Most of the rest of the keyboard and other helpful actions are included as modifier gestures, such as: shift: close right eye command: close left eye arrow up/down: raise left/right eyebrow … Watch the video where he does a coding interview for a big tech company using no keyboard. Nick #3: Is Google’s LaMDA Model Sentient? authored by Richard Luscombe (The Guardian) The Google engineer who thinks the company’s AI has come to life Transcript of conversation Brian #4: richbench Also from Anthony “A little Python benchmarking tool.” Give it a list of (first_func, second_func, “label”), and it times them and prints out a comparison. Simple and awesome. def sort_seven(): """Sort a list of seven items""" for _ in range(10_000): sorted([3,2,4,5,1,5,3]) def sort_three(): """Sort a list of three items""" for _ in range(10_000): sorted([3,2,4]) __benchmarks__ = [ (sort_seven, sort_three, "Sorting 3 items instead of 7") ] Michael #5: typeguard A run-time type checker for Python Three principal ways to do type checking are provided, each with its pros and cons: Manually with function calls @typechecked decorator import hook (typeguard.importhook.install_import_hook()) Example: @typechecked def some_function(a: int, b: float, c: str, *args: str) -> bool: ... return retval Nick #6: CustomTkinter A modern and customizable python UI-library based on Tkinter. Extras Michael: OpenSSF Funds Python and Eclipse Foundations - OpenSSF’s Alpha-Omega Project has committed $400K to the Python Software Foundation (PSF), in order to create a new role which will provide security expertise for Python, the Python Package Index (PyPI), and the rest of the Python ecosystem, as well as funding a security audit. (via Python Weekly) Nick: Terms of Service Didn’t Read - Terms of Service; Didn't Read” (short: ToS;DR) is a young project started in June 2012 to help fix the “biggest lie on the web”: almost no one really reads the terms of service we agree to all the time. Joke: Serverless A DevOps approach to COVID-19
#289 Textinator is coming for your text, wherever it is
Watch the live stream: Watch on YouTube About the show Sponsored by us! Support our work through: Our courses at Talk Python Training Test & Code Podcast Patreon Supporters Special guest: Gina Häußge, creator & maintainer of OctoPrint Michael #1: beanita Local MongoDB-like database prepared to work with Beanie ODM So, you know Beanie - Pydantic + async + MongoDB And you know Mongita - Mongita is to MongoDB as SQLite is to SQL Beanita lets you use Beanie, but against Mongita rather than a server-based MongoDB server Brian #2: The Good Research Code Handbook Patrick J Mineault “for grad students, postdocs and PIs (principle investigator) who do a lot of programming as part of their research.” lessons setup git, virtual environments, project layout, packaging, cookie cutter style style guides, keeping things clean coding separating concerns, separating pure functions and those with side effects, pythonic-ness testing unit testing, testing with side effects, … (incorrect definition of end-to-end tests, but a good job at covering the other bits) documentation comments, tests, docstrings, README.md, usage docs, tutorials, websites documenting pipelines and projects social aspects various reviews, pairing, open source, community sample project extras testing example good tools to use Gina #3: CadQuery Python lib to do build parametric 3D CAD models Can output STL, STEP, AMF, SVG and some more Uses same geometry kernel as FreeCAD (OpenCascade) Also available: desktop editor, Jupyter extension, CLI Would recommend the Jupyter extension, the app seems a bit behind latest development Jupyter extension is easy to set up on Docker and comes with a nice 3D preview pane Was able to create a basic parametric design of an insert for an assortment box easily Python 3.8+, not yet 3.11, OpenCascade related Michael #4: Textinator Like TextSniper, but in Python Simple MacOS StatusBar / Menu Bar app to automatically detect text in screenshots Built with RUMPS: Ridiculously Uncomplicated macOS Python Statusbar apps Take a screenshot of a region of the screen using ⌘ + ⇧ + 4 (Cmd + Shift + 4). The app will automatically detect any text in the screenshot and copy it to your clipboard. How Textinator Works At startup, Textinator starts a persistent NSMetadataQuery Spotlight query (using the pyobjc Python-to-Objective-C bridge) to detect when a new screenshot is created. When the user creates screenshot, the NSMetadataQuery query is fired and Textinator performs text detection using a Vision VNRecognizeTextRequest call. Brian #5: Handling Concurrency Without Locks "How to not let concurrency cripple your system” Haki Benita “…common concurrency challenges and how to overcome them with minimal locking.” Starts with a Django web app A url shortener that generates a unique short url and stores the result in a database so it doesn’t get re-used. Discussions of collision with two users checking, then storing keys at the same time. locking problems in general utilizing database ability to make sure some items are unique, in this case PostgreSQL updating your code to take advantage of database constraints support to allow you to do less locking within your code Gina #6: TatSu Generates parsers from EBNF grammars (or ANTLR) Can compile the model (similar to regex) for quick reuse or generate python source Many examples provided Active development, Python 3.10+ Extras Michael: Back on 285 we spoke about PEP 690. Now there is a proper blog post about it. Expedited release of Python3.11.0b3 - Due to a known incompatibility with pytest and the previous beta release (Python 3.11.0b2) and after some deliberation, Python release team have decided to do an expedited release of Python 3.11.0b3 so the community can continue testing their packages with pytest and therefore testing the betas as expected. (via Python Weekly) Kagi search via Daniel Hjertholm Not really python related, but if I know Michael right, he'll love the new completely ad free and privacy-respecting search engine kagi.com. I've used kagi.com since their public beta launched, mainly to search for solutions to Python issues at work. The results are way better than DuckDuckGo's results, and even better than Googles! Love the Programming-lens and the ability to up/down prioritize domains in the results. Their FAQ explains everything you need to know: https://kagi.com/faq Looks great but not sure about the pricing justification (32 sec of compute = $1), that’s either 837x more than all of Talk Python + Python Bytes or more than 6,700x more than just one of our sites/services. (We spend about $100/mo on 8 servers.) But they may be buying results from Google and Bing, and that could be the cost. Here's a short interview with the man who started kagi. Gina: rdserialtool: Reads out low-cost USB power monitors (UM24C, UM25C, UM34C) via BLE/pybluez. Amazing if you need to monitor the power consumption/voltage/current of some embedded electronics on a budget. Helped me solve a very much OctoPrint development specific problem. Python 3.4+ nodejs-bin: by Sam Willis: https://twitter.com/samwillis/status/1537787836119793667 Install nodejs via pypi/as dependency, still very much an Alpha but looks promising Makes it easier to obtain a full stack environment Very interesting for end to end testing with JS based tooling, or packaging a frontend with your Python app See also nodeenv, which does a similar thing, but with additional steps Joke: Rejected Github Badges
#288 Performance benchmarks for Python 3.11 are amazing
Watch the live stream: Watch on YouTube About the show Sponsored by us! Support our work through: Our courses at Talk Python Training Test & Code Podcast Patreon Supporters Brian #1: Polars: Lightning-fast DataFrame library for Rust and Python Suggested by a several listeners “Polars is a blazingly fast DataFrames library implemented in Rust using Apache Arrow Columnar Format as memory model. Lazy | eager execution Multi-threaded SIMD (Single Instruction/Multiple Data) Query optimization Powerful expression API Rust | Python | ...” Python API syntax set up to allow parallel and execution while sidestepping GIL issues, for both lazy and eager use cases. From the docs: Do not kill parallelization The syntax is very functional and pipeline-esque: import polars as pl q = ( pl.scan_csv("iris.csv") .filter(pl.col("sepal_length") > 5) .groupby("species") .agg(pl.all().sum()) ) df = q.collect() Polars User Guide is excellent and looks like it’s entirely written with Python examples. Includes a 30 min intro video from PyData Global 2021 Michael #2: PSF Survey is out Have a look, their page summarizes it better than my bullet points will. Brian #3: Gin Config: a lightweight configuration framework for Python Found through Vincent D. Warmerdam’s excellent intro videos on gin on calmcode.io Quickly make parts of your code configurable through a configuration file with the @gin.configurable decorator. It’s in interesting take on config files. (Example from Vincent) # simulate.py @gin.configurable def simulate(n_samples): ... # config.py simulate.n_samples = 100 You can specify: required settings: def simulate(n_samples=gin.REQUIRED)` blacklisted settings: @gin.configurable(blacklist=["n_samples"]) external configurations (specify values to functions your code is calling) can also references to other functions: dnn.activation_fn = @tf.nn.tanh Documentation suggests that it is especially useful for machine learning. From motivation section: “Modern ML experiments require configuring a dizzying array of hyperparameters, ranging from small details like learning rates or thresholds all the way to parameters affecting the model architecture. Many choices for representing such configuration (proto buffers, tf.HParams, ParameterContainer, ConfigDict) require that model and experiment parameters are duplicated: at least once in the code where they are defined and used, and again when declaring the set of configurable hyperparameters. Gin provides a lightweight dependency injection driven approach to configuring experiments in a reliable and transparent fashion. It allows functions or classes to be annotated as @gin.configurable, which enables setting their parameters via a simple config file using a clear and powerful syntax. This approach reduces configuration maintenance, while making experiment configuration transparent and easily repeatable.” Michael #4: Performance benchmarks for Python 3.11 are amazing via Eduardo Orochena Performance may be the biggest feature of all Python 3.11 has task groups in asyncio fine-grained error locations in tracebacks the self-type to return an instance of their class The "Faster CPython Project" to speed-up the reference implementation. See my interview with Guido and Mark: talkpython.fm/339 Python 3.11 is 10~60% faster than Python 3.10 according to the official figures And a 1.22x speed-up with their standard benchmark suite. Arriving as stable until October Extras Michael: Python 3.10.5 is available (changelog) Raycast (vs Spotlight) e.g. CMD+Space => pypi search: Joke: Why wouldn't you choose a parrot for your next application
#287 Surprising ways to use Jupyter Notebooks
Watch the live stream: Watch on YouTube About the show Sponsored by us! Support our work through: Our courses at Talk Python Training Test & Code Podcast Patreon Supporters Michael #1: auto-py-to-exe Converts .py to .exe using a simple graphical interface A good candidate to install via pipx For me, just point it at the top level app.py file and click go Can add icons, etc. Got a .app version and CLI version (I think 😉 ) Required brew install python-tk to get tkinter on my mac I tested it against my URLify app. Oddly, only ran on Python 3.9 but not 3.10 Brian #2: 8 surprising ways how to use Jupyter Notebook by Aleksandra Płońska, Piotr Płoński Fun romp through ways you can use and abuse notebooks package development web app slides book blog report dashboard REST API Michael #3: piptrends by Tankala Ashok Use piptrends.com for comparing python packages downloads and GitHub Statistics. Whenever doing research which python package, check multiple places to finalize it so thought of putting all those things in a single place. Inspired by npmtends.com. Brian #4: Is it a class or a function? It's a callable! by Trey Hunner It’s kinda hard to tell in Python. Actually, impossible to tell from staring at the calling code. “Of the 69 “built-in functions” listed in the Python Built-In Functions page, only 42 are actually implemented as functions: 26 are classes and 1 (help) is an instance of a callable class. Of the 26 classes among those built-in “functions”, four were actually functions in Python 2 (the now-lazy map, filter, range, and zip) but have since become classes. The Python built-ins and the standard library are both full of maybe-functions-maybe-classes.” len - yep, that’s a function zip - that’s a class reversed, enumerate, range, and filter “functions” are all classes. But callable classes. Cool discussion of callable objects partials, itemgetters, iterators, generators, factory functions … Extras Brian: What’s in which Python - Ned Batchelder brief bullet list of a few memorable changes in versions 2.1 through 3.11 Michael: Orion Browser via Dan Bader PSF 2021 Survey Results are out (full analysis next week) Joke: async problems
#286 Unreasonable f-strings
Watch the live stream: Watch on YouTube About the show Sponsored by us! Support our work through: Our courses at Talk Python Training Test & Code Podcast Patreon Supporters Brian #1: The Python GIL: Past, Present, and Future Bary Warsaw and Paweł Polewicz Michael #2: Announcing the PyOxy Python Runner PyOxy is all of the following: An executable program used for running Python interpreters. A single file and highly portable (C)Python distribution. An alternative python driver providing more control over the interpreter than what python itself provides. A way to make some of PyOxidizer's technology more broadly available without using PyOxidizer. PyOxidizer is often used to generate binaries embedding a Python interpreter and a custom Python application. However, its configuration files support additional functionality, such as the ability to produce Windows MSI installers, macOS application bundles, and more. The pyoxy executable also embeds a copy of the Python standard library and imports it from memory using the oxidized_importer Python extension module. Brian #3: The unreasonable effectiveness of f-strings and re.VERBOSE Michael #4: PyCharm PR Management Really nice but not very discoverable Not covered in the docs, but super useful. Available in pro and free community edition Steps Open a project that has an associated github git repo If the GitHub repo has a PR, you’ll see it in the Pull Requests tab. Browse the PRs, and open them for details There you can see the comments, close or merge it, and more Most importantly, check it out to see how it works Extras Brian: Pandas Tutor: Using Pyodide to Teach Data Science at Scale Michael: Python + pyscript + WebAssembly: Python Web Apps, Running Locally with pyscript video is out And an iOS Python Apps video too Joke: Losing an orm!
#285 Where we talk about UIs and Python
Watch the live stream: Watch on YouTube About the show Sponsored: RedHat: Compiler Podcast Special guests Mark Little Ben Cosby Michael #1: libgravatar A library that provides a Python 3 interface to the Gravatar APIs. If you have users and want to show some sort of an image, Gravatar is OK PyPI uses this for example (gravatar, not necessarily this lib) Usage: >>> g = Gravatar('myemailaddress@example.com') >>> g.get_image() 'https://www.gravatar.com/avatar/0bc83cb571cd1c50ba6f3e8a78ef1346' Brian #2: JSON to Pydantic Converter Suggested by Chun Ly, “this awesome JSON to @samuel_colvin's pydantic is so useful. It literally saved me days of work with a complex nested JSON schema.“ “JSON to Pydantic is a tool that lets you convert JSON objects into Pydantic models.” It’s a live site, where you can plop JSON on one the left, and Pydantic models show up on the right. There’s a couple options: Specify every field as Optional Alias camelCase fields as snake_case It’s also an open source project, built with FastAPI, Create React App, and a project called datamodel-code-generator. Mark #3: tailwindcss, tailwindui Not python, but helpful for web UI and open source business model example tailwindcss generates CSS Used on the Lexchart app Benefits of tailwindcss and tailwindui: Just-in-Time makes it fast. Output includes only classes used for the project. Stand on shoulders of design thinking from Steve Schoger and Adam Wathan. See also refactoingui.com. Use in current projects without CSS conflicts. Custom namespace with prefix in tailwind.config.js. Bonus: custom namespace prefixes work with the tailwind plug-ins for VS Code and PyCharm. Works well with template engines like, Chameleon. We use tailwind for our app UI. Toolbar template example. Another example of docs and tutorials being a strategic business asset. Resources tailwindcss.com tailwindlabs on YouTube, great tutorials from Simon at Tailwind Beginner friendly tutorials: Thirus, example of tailwind install methods Michael #4: PEP 690 – Lazy Imports From Itamar Discussion at https://discuss.python.org/t/pep-690-lazy-imports/15474 PEP proposes a feature to transparently defer the execution of imported modules until the moment when an imported object is used. PEP 8 says imports go a the top, that means you pay the full price of importing code This means that importing the main module of a program typically results in an immediate cascade of imports of most or all of the modules that may ever be needed by the program. Lazy imports also mostly eliminate the risk of import cycles or crashes. The implementation in this PEP has already demonstrated startup time improvements up to 70% and memory-use reductions up to 40% on real-world Python CLIs. Brian #5: Two small items pytest-rich Suggested by Brian Skinn Created by Bruno Oliveira as a proof of concept pytest + rich, what’s not to love? Now we just need a maintainer or two or three…. Embedding images in GitHub README Suggested by Henrik Finsberg Video by Anthony Sottile This is WITHOUT putting the image in the repo. Upload or drop an image to an issue comment. Don’t save the comment, just wait for GitHub to upload it to their CDN. GH will add a markdown link in the comment text box with a link to the now uploaded image. Now you can use that image in a README file. You can do the same while editing the README in the online editor. Ben #6: pyotp A library for generating and verifying one-time passwords (OTP). Helpful for implementing multi-factor authentication (MFA) in web applications. Supports HMAC-based one-time passwords (HOTP) and time-based one-time passwords (TOTP). While HOTP delivered via SMS text messages is a common approach to implementing MFA, SMS is not really secure. TOTP using an authenticator app on the user’s device such as Google Authenticator or Microsoft Authenticator is more secure, fairly easy to implement, and free (no SMS messaging fees and multiple free authenticator apps available for users). TOTP works best by making a QR code available to simplify the setup for the user in their authenticator app. Lots of easy to implement QR code generators to choose from (qrcode is a popular one if you use javascript on the front end). TOTP quick reference: import pyotp def generate_shared_secret(): # securely store this shared secret with user account data return pyotp.random_base32() def generate_provisioning_uri(secret, email): # generate uri for a QR code from the user's shared secret and email address return pyotp.totp.TOTP(secret).provisioning_uri(name=email, issuer_name='YourApp') def verify_otp(secret, otp): # verify user's one-time password entry with their shared secret totp = pyotp.TOTP(secret) return totp.verify(otp) Extras Brian: PyConUS 2022 videos now up A few more Python related extensions for VSCode black, pylint, isort, and Jupyter PowerToys Work has begun on a pytest course Saying this in public to inspire me to finish it. No ETA yet Sad Python Girls Club podcast Michael: PyTorch M1 Mission Encodable PWAs and pyscript Michael's now released pyscript PWA YouTube video cal.com (open source calendly) Supabase (open source Firebase) Joke: Beginner problems
#284 Spicy git for Engineers
Watch the live stream: Watch on YouTube About the show Sponsored by us! Support our work through: Our courses at Talk Python Training Test & Code Podcast Patreon Supporters Brian #1:distinctipy “distinctipy is a lightweight python package providing functions to generate colours that are visually distinct from one another.” Small, focused tool, but really cool. Say you need to plot a dynamic number of lines. Why not let distinctipy pick colors for you that will be distinct? Also can display the color swatches. Some example palettes here: https://github.com/alan-turing-institute/distinctipy/tree/main/examples from distinctipy import distinctipy # number of colours to generate N = 36 # generate N visually distinct colours colors = distinctipy.get_colors(N) # display the colours distinctipy.color_swatch(colors) Michael #2: Soda SQL Soda SQL is a free, open-source command-line tool. It utilizes user-defined input to prepare SQL queries that run tests on dataset in a data source to find invalid, missing, or unexpected data. Looks good for data pipelines and other CI/CD work! Daniel #3: Python in Nature There’s a review article from Sept 2020 on array programming with NumPy in the research journal Nature. For reference, in grad school we had a fancy paper on quantum entanglement that got rejected from Nature Communications, a sub-journal to Nature. Nature is hard to get into. List of authors includes Travis Oliphant who started NumPy. Covers NumPy as the foundation, building up to specialized libraries like QuTiP for quantum computing. If you search “Python” on their site, many papers come up. Interesting to see their take on publishing software work. Brian #4: Supercharging GitHub Actions with Job Summaries From a tweet by Simon Willison and an article: GH Actions job summaries Also, Ned Batchelder is using it for Coverage reports “You can now output and group custom Markdown content on the Actions run summary page.” “Custom Markdown content can be used for a variety of creative purposes, such as: Aggregating and displaying test results Generating reports Custom output independent of logs” Coverage.py example: - name: "Create summary" run: | echo '### Total coverage: ${{ env.total }}%' >> $GITHUB_STEP_SUMMARY echo '[${{ env.url }}](${{ env.url }})' >> $GITHUB_STEP_SUMMARY Michael #5:Language Summit is write up out via Itamar, by Alex Waygood Python without the GIL: A talk by Sam Gross Reaching a per-interpreter GIL: A talk by Eric Snow The "Faster CPython" project: 3.12 and beyond: A talk by Mark Shannon WebAssembly: Python in the browser and beyond: A talk by Christian Heimes F-strings in the grammar: A talk by Pablo Galindo Salgado Cinder Async Optimisations: A talk by Itamar Ostricher The issue and PR backlog: A talk by Irit Katriel The path forward for immortal objects: A talk by Eddie Elizondo and Eric Snow Lightning talks, featuring short presentations by Carl Meyer, Thomas Wouters, Kevin Modzelewski, Samuel Colvin and Larry Hastings Daniel #6:AllSpice is Git for EEs Software engineers have Git/SVN/Mercurial/etc None of the other engineering disciplines (mechanical, electrical, optical, etc), have it nearly as good. Altium has their Vault and “365,” but there’s nothing with a Git-like UX. Supports version history, diffs, all the things you expect. Even self-hosting and a Gov Cloud version. “Bring your workflow to the 21st century, finally.” Extras Brian: Will McGugan talks about Rich, Textual, and Textualize on Test & Code 188 Also 3 other episodes since last week. (I have a backlog I’m working through.) Michael: Power On-Xbox Documentary | Full Movie The 4 Reasons To Branch with Git - Illustrated Examples with Python A Python spotting - via Jason Pecor 2022 StackOverflow Developer Survey is live, via Brian TextSniper macOS App PandasTutor on webassembly Daniel: I know Adafruit’s a household name, shout-out to Sparkfun, Seeed Studio, OpenMV, and other companies in the field. Joke: A little awkward
#283 The sports episode
Watch the live stream: Watch on YouTube About the show Sponsored: RedHat: Compiler Podcast Special guest: Tonya Sims Michael #1: Pathy: a Path interface for local and cloud bucket storage via Spencer Pathy is a python package (with type annotations) for working with Cloud Bucket storage providers using a pathlib interface. It provides an easy-to-use API bundled with a CLI app for basic file operations between local files and remote buckets. It enables a smooth developer experience by letting developers work against the local file system during development and only switch over to live APIs for deployment. Also has optional local file caching. From Spenser The really cool function is "Pathy.fluid" which can take any type of local, GCS, or S3 path string and then just give you back a Path object that you can interact with agnostic of what platform it was. So this has worked amazingly for me in local testing since i can just change the file path from the "s3://bucket/path" that i use in prod to a local "test_dir/path" and it works automatically. Brian #2: Robyn “Robyn is a fast, high-performance Python web framework with a Rust runtime.” Hello, Robyn! - intro article docs, repo Neat things doesn’t need WSGI or ASGI async very Flask-like Early, so still needs some TLC docs, etc. getting started and demo apps would be good. Tonya #3: Python package 'nba_api' is a package to access data for NBA.com This package is maintained by Swar Patel API Client package for NBA.com, more accessible endpoints, and better documentation The NBA.com API's are not well documented and change frequently (player traded, injured, retired, points per game, stats, etc) The nba_api package has tons of features: The nba_api starts with static data on players and teams (Full name, team name, etc). Each player and Team has an id. Can get game data from the playergamelog API endpoint The package also has many different API endpoints that it can hit by passing in features from the static data to the API endpoints as parameters Michael #4: Termshot From Jay Miller Creates screenshots based on terminal command output Just run termshot YOUR_CMD or termshot --show-cmd -- python program.py Even termshot /bin/zsh for full interactive “recording” Example I made: Brian #5: When Python can’t thread: a deep-dive into the GIL’s impact Itamar Turner-Trauring Building a mental model of the GIL using profiler graphs of simple two thread applications. The graphs really help a lot to see when the CPU is active or waiting on each thread. Tonya #6: Sportsipy: A free sports API written for python Free python API that pulls the stats from www.sports-reference.com sports-reference.com - great website for getting sports stats for professional sports(NBA, NFL, NHL, MLB, college sports) Looks like an HTML website for the 90s - great for scraping (email site owners) You can get API queries for every sport (North American sports) like the list of teams for that sport, the date and time of a game, the total number of wins for a team during the season, and many more team-related metrics. You can also get stats from players and box scores - so you can build cool stuff around how a team performed during a game or during a season. Extras Michael: Python 3.11.0 beta 1 is out Test with GitHub Actions against Python 3.11 Joke:Finding my family
#282 Don't Embarrass Me in Front of The Wizards
Watch the live stream: Watch on YouTube About the show Sponsored by us! Support our work through: Our courses at Talk Python Training Test & Code Podcast Patreon Supporters Brian #1: pyscript Python in the browser, from Anaconda. repo here Announced at PyConUS “During a keynote speech at PyCon US 2022, Anaconda’s CEO Peter Wang unveiled quite a surprising project — PyScript. It is a JavaScript framework that allows users to create Python applications in the browser using a mix of Python and standard HTML. The project’s ultimate goal is to allow a much wider audience (for example, front-end developers) to benefit from the power of Python and its various libraries (statistical, ML/DL, etc.).” from a nice article on it, PyScript — unleash the power of Python in your browser PyScript is built on Pyodide, which is a port of CPython based on WebAssembly. Demos are cool. Note included in README: “This is an extremely experimental project, so expect things to break!” Michael #2: Memray from Bloomberg Memray is a memory profiler for Python. It can track memory allocations in Python code native extension modules the Python interpreter itself Works both via CLI and focused app calls Memray can help with the following problems: Analyze allocations in applications to help discover the cause of high memory usage. Find memory leaks. Find hotspots in code which cause a lot of allocations. Notable features: 🕵️♀️ Traces every function call so it can accurately represent the call stack, unlike sampling profilers. ℭ Also handles native calls in C/C++ libraries so the entire call stack is present in the results. 🏎 Blazing fast! Profiling causes minimal slowdown in the application. Tracking native code is somewhat slower, but this can be enabled or disabled on demand. 📈 It can generate various reports about the collected memory usage data, like flame graphs. 🧵 Works with Python threads. 👽🧵 Works with native-threads (e.g. C++ threads in native extensions) Has a live view in the terminal. Linux only Brian #3: pytest-parallel I’ve often sped up tests that can be run in parallel by using -n from pytest-xdist. I was recommending this to someone on Twitter, and Bruno Oliviera suggested a couple of alternatives. One was pytest-parallel, so I gave it a try. pytest-xdist runs using multiprocessing pytest-parallel uses both multiprocessing and multithreading. This is especially useful for test suites containing threadsafe tests. That is, mostly, pure software tests. Lots of unit tests are like this. System tests are often not. Use --workers flag for multiple processors, --workers auto works great. Use --tests-per-worker for multi-threading. --tesst-per-worker auto let’s it pick. Very cool alternative to xdist. - Michael #4: Pooch: A friend for data files via via Matthew Fieckert Just want to download a file without messing with requests and urllib? Who is it for? Scientists/researchers/developers looking to simply download a file. Pooch makes it easy to download a file (one function call). On top of that, it also comes with some bonus features: Download and cache your data files locally (so it’s only downloaded once). Make sure everyone running the code has the same version of the data files by verifying cryptographic hashes. Multiple download protocols HTTP/FTP/SFTP and basic authentication. Download from Digital Object Identifiers (DOIs) issued by repositories like figshare and Zenodo. Built-in utilities to unzip/decompress files upon download file_path = pooch.retrieve(url) Extras Michael: New course! Up and Running with Git - A Pragmatic, UI-based Introduction. Joke: Don’t embarrass me in front of the wizards Michael’s crashing github is embarrassing him in front of the wizards!